class: center, middle, inverse, title-slide # AI Technologies in Endoscopy ## IRCAD Masterclass: Flexible Endoscopy ### Thomas Ward, MD
Surgical AI and Innovation Laboratory
Massachusetts General Hospital ### May 4, 2021 --- # Disclosures Research support from the Olympus Corporation. --- class: center, middle # Computer Vision (CV) -- ## Subfield of AI --- background-image: url(imgs/cnn.svg) # Computer Vision:<br/>Convolutional Neural Networks --- class: center, middle # CV for Diagnosis in Endoscopy ## Lesion identification --- class: center, middle # Upper GI Neoplasia Diagnosis 90% average accuracy across 19 studies for diagnosis of upper gastrointestinal neoplasias¹ .pull-left[.footnote[¹Arribas J, et al. Gut 2020;0:1–11. [doi:10.1136/gutjnl-2020-321922](https://doi.org/10.1136/gutjnl-2020-321922)]] --- # Barrett's Esophagus Diagnosis -- .pull-left[ - 1,704 images of Barrett's esophagus (BE) from 4 centers - Trained to 1. Classify lesions as dysplastic versus not 2. Segment lesion, generating a heatmap of dysplatic areas and indiciator for best place to biopsy - Evaluated on two external validation sets ] .pull-right[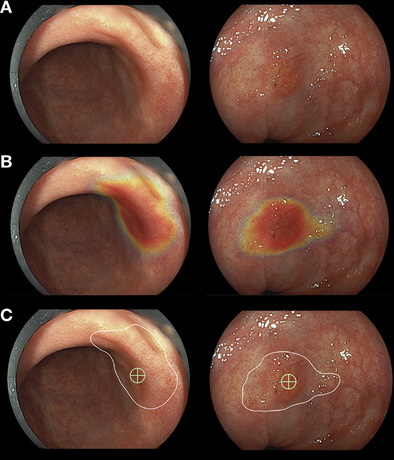] .pull-left[.footnote[¹de Groof A, et al. Gastroenterology 2020;158:915-929. [doi:10.1053/j.gastro.2019.11.030](https://doi.org/10.1053/j.gastro.2019.11.030)]] --- background-image: url(imgs/be-perf.jpg) # Dysplastic BE Diagnosis: Performance CV model versus 53 endoscopics on an external dataset with only *subtle* BE .footnote[¹de Groof A, et al. Gastroenterology 2020;158:915-929. [doi:10.1053/j.gastro.2019.11.030](https://doi.org/10.1053/j.gastro.2019.11.030)] --- # Lower GI Diagnostics:<br/>Real-time detection -- Prospective trial of 1,058 patients randomized to colonoscopy with or without real-time polyp detection system. 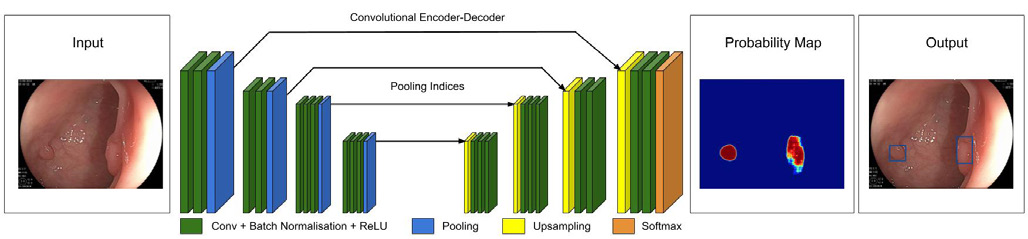 .footnote[¹Wang P, et al. Gut 2019;68:1813–1819. [doi:10.1136/gutjnl-2018-317500](https://doi.org/10.1136/gutjnl-2018-317500)] --- # Lower GI Diagnostics:<br/>Real-time detection .footnote[¹Wang P, et al. Gut 2019;68:1813–1819. [doi:10.1136/gutjnl-2018-317500](https://doi.org/10.1136/gutjnl-2018-317500)] ## All polyps - With system: 498 - Without: 269 -- ## Adenoma-detection rate - With system: 29.1% - Without: 20.3% -- ## Advanced adenomas detected - With system: 17 - Without: 16 --- # Lower GI Diagnostics:<br/>Real-time detection .footnote[¹Wang P, et al. Gut 2019;68:1813–1819. [doi:10.1136/gutjnl-2018-317500](https://doi.org/10.1136/gutjnl-2018-317500)] ## False alarms - 39 total - **0.075** per colonoscopy -- ## Withdrawal time (excluding biopsy time) - Without system: 6.07 minutes - With system: 6.18 minutes --- class:center, middle # CV for Diagnosis in Endoscopy ## Blind spot prevention --- # Blind spot prevention: Upper GI<br/>WISENSE .footnote[¹Wu L, Zhang J, Zhou W et al. Gut 2019;68:2161-2169. [doi:10.1136/gutjnl-2018-317366](https://doi.org/10.1136/gutjnl-2018-317366)] -- ## Design - Single-center randomized trial - 324 patients undergoing routine esophagogastroduodenoscopy (EGD) - Randomized to: 1. Normal EGD 2. EGD with WISENSE system -- ## WISENSE CV Model - Blind spot prevention system - Updates clinican, in real-time, to anatomical sites examined - Need to examine 26 different anatomical areas to receive a perfect score --- background-image: url(imgs/wisense.jpg) # WISENSE: Real-time feedback .footnote[¹Wu L, Zhang J, Zhou W et al. Gut 2019;68:2161-2169. [doi:10.1136/gutjnl-2018-317366](https://doi.org/10.1136/gutjnl-2018-317366)] --- # WISENSE: Results .footnote[¹Wu L, Zhang J, Zhou W et al. Gut 2019;68:2161-2169. [doi:10.1136/gutjnl-2018-317366](https://doi.org/10.1136/gutjnl-2018-317366)] -- ## Blind spot rate - Control group: 22.46% - WISENSE group: 5.86% - Mean difference: -15.39% (95% CI -11.54, -19.23) -- ## Procedure time - Control group: 4.24 min - WISENSE group: 5.03 min --- class: center, middle # Blind spot prevention: Lower GI -- ## C2D2 Colonoscopy Coverage Deficiency via Depth .pull-left[.footnote[Freeman D et al. IEEE Transactions on Medical Imaging 2020;39;11:3451-3462. [doi:10.1109/TMI.2020.2994221](https://doi.org/10.1109/TMI.2020.2994221)]] --- background-image: url(imgs/coverage.jpg) # C2D2: Coverage Coverage = Orange/Green .footnote[Freeman D et al. IEEE Transactions on Medical Imaging 2020;39;11:3451-3462. [doi:10.1109/TMI.2020.2994221](https://doi.org/10.1109/TMI.2020.2994221)] --- background-image: url(imgs/colon.jpg) # C2D2: Synthetic Data .footnote[Freeman D et al. IEEE Transactions on Medical Imaging 2020;39;11:3451-3462. [doi:10.1109/TMI.2020.2994221](https://doi.org/10.1109/TMI.2020.2994221)] --- background-image: url(imgs/physician-vs-c2d2.jpg) # C2D2: Why synthetic data? .footnote[Freeman D et al. IEEE Transactions on Medical Imaging 2020;39;11:3451-3462. [doi:10.1109/TMI.2020.2994221](https://doi.org/10.1109/TMI.2020.2994221)] -- 1. Know ground truth with 100% certainty -- 2. Physicians are not great at judging coverage: --- background-image: url(imgs/real-colon.jpg) # C2D2: Performance .footnote[Freeman D et al. IEEE Transactions on Medical Imaging 2020;39;11:3451-3462. [doi:10.1109/TMI.2020.2994221](https://doi.org/10.1109/TMI.2020.2994221)] -- Physicians agreed with C2D2's prediction on actual patient video clips 93% of the time. --- class:center, middle # Procedure ## POEM --- # POEM .pull-left[ - Trained CV model to recognize surgical phases - 50 full-length POEM videos from two hospitals. One in the US and one in Japan - 87.6% accuracy ] .pull-right[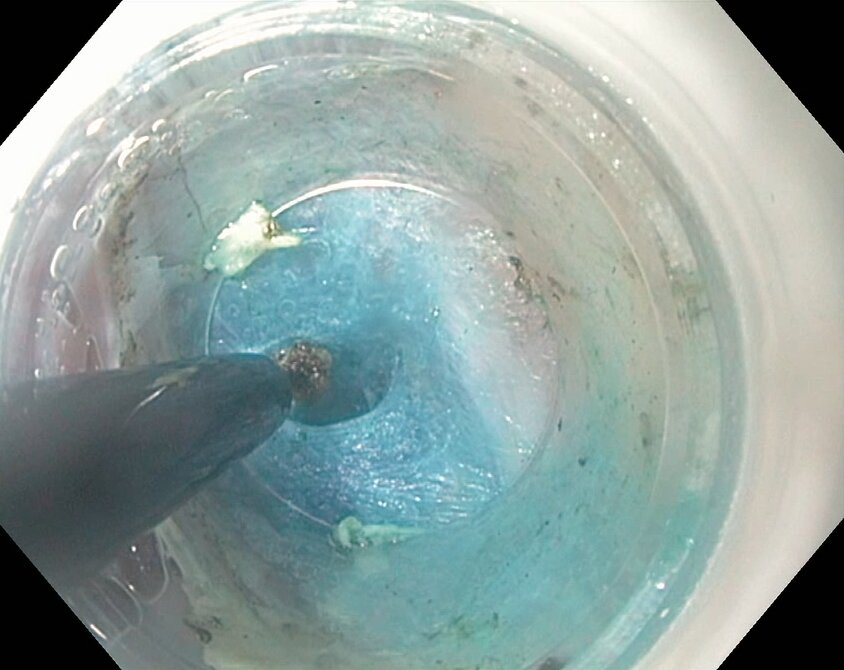] .pull-left[.footnote[Ward T, et al. Surg Endosc 2020. [doi:10.1007/s00464-020-07833-9](https://doi.org/10.1007/s00464-020-07833-9)]] --- background-image: url(imgs/poem3.png) # Surgical Fingerprints .pull-left[.footnote[Ward T, et al. Surg Endosc 2020. [doi:10.1007/s00464-020-07833-9](https://doi.org/10.1007/s00464-020-07833-9)]] --- # Conclusions -- ## AI/CV in Diagnostics -- - Accurate classification of neoplastic lesions -- - Blind spot warning systems to ensure complete inspection -- ## AI/CV in Procedures -- - Accurate identification of procedure phase in POEM -- ## Moving forward -- - Larger, more diverse, datasets from multiple institutions and countries - Continue to transition from theoretical papers to actual patient applications --- class: center, middle # Questions? <svg xmlns="http://www.w3.org/2000/svg" viewBox="0 0 512 512" class="rfa" style="height:0.75em;fill:currentColor;position:relative;"><path d="M476 3.2L12.5 270.6c-18.1 10.4-15.8 35.6 2.2 43.2L121 358.4l287.3-253.2c5.5-4.9 13.3 2.6 8.6 8.3L176 407v80.5c0 23.6 28.5 32.9 42.5 15.8L282 426l124.6 52.2c14.2 6 30.4-2.9 33-18.2l72-432C515 7.8 493.3-6.8 476 3.2z"/></svg> [tmward@mgh.harvard.edu](mailto:tmward@mgh.harvard.edu) <svg xmlns="http://www.w3.org/2000/svg" viewBox="0 0 496 512" class="rfa" style="height:0.75em;fill:currentColor;position:relative;"><path d="M165.9 397.4c0 2-2.3 3.6-5.2 3.6-3.3.3-5.6-1.3-5.6-3.6 0-2 2.3-3.6 5.2-3.6 3-.3 5.6 1.3 5.6 3.6zm-31.1-4.5c-.7 2 1.3 4.3 4.3 4.9 2.6 1 5.6 0 6.2-2s-1.3-4.3-4.3-5.2c-2.6-.7-5.5.3-6.2 2.3zm44.2-1.7c-2.9.7-4.9 2.6-4.6 4.9.3 2 2.9 3.3 5.9 2.6 2.9-.7 4.9-2.6 4.6-4.6-.3-1.9-3-3.2-5.9-2.9zM244.8 8C106.1 8 0 113.3 0 252c0 110.9 69.8 205.8 169.5 239.2 12.8 2.3 17.3-5.6 17.3-12.1 0-6.2-.3-40.4-.3-61.4 0 0-70 15-84.7-29.8 0 0-11.4-29.1-27.8-36.6 0 0-22.9-15.7 1.6-15.4 0 0 24.9 2 38.6 25.8 21.9 38.6 58.6 27.5 72.9 20.9 2.3-16 8.8-27.1 16-33.7-55.9-6.2-112.3-14.3-112.3-110.5 0-27.5 7.6-41.3 23.6-58.9-2.6-6.5-11.1-33.3 2.6-67.9 20.9-6.5 69 27 69 27 20-5.6 41.5-8.5 62.8-8.5s42.8 2.9 62.8 8.5c0 0 48.1-33.6 69-27 13.7 34.7 5.2 61.4 2.6 67.9 16 17.7 25.8 31.5 25.8 58.9 0 96.5-58.9 104.2-114.8 110.5 9.2 7.9 17 22.9 17 46.4 0 33.7-.3 75.4-.3 83.6 0 6.5 4.6 14.4 17.3 12.1C428.2 457.8 496 362.9 496 252 496 113.3 383.5 8 244.8 8zM97.2 352.9c-1.3 1-1 3.3.7 5.2 1.6 1.6 3.9 2.3 5.2 1 1.3-1 1-3.3-.7-5.2-1.6-1.6-3.9-2.3-5.2-1zm-10.8-8.1c-.7 1.3.3 2.9 2.3 3.9 1.6 1 3.6.7 4.3-.7.7-1.3-.3-2.9-2.3-3.9-2-.6-3.6-.3-4.3.7zm32.4 35.6c-1.6 1.3-1 4.3 1.3 6.2 2.3 2.3 5.2 2.6 6.5 1 1.3-1.3.7-4.3-1.3-6.2-2.2-2.3-5.2-2.6-6.5-1zm-11.4-14.7c-1.6 1-1.6 3.6 0 5.9 1.6 2.3 4.3 3.3 5.6 2.3 1.6-1.3 1.6-3.9 0-6.2-1.4-2.3-4-3.3-5.6-2z"/></svg> [@tmward](https://github.com/tmward) <svg xmlns="http://www.w3.org/2000/svg" viewBox="0 0 512 512" class="rfa" style="height:0.75em;fill:currentColor;position:relative;"><path d="M459.37 151.716c.325 4.548.325 9.097.325 13.645 0 138.72-105.583 298.558-298.558 298.558-59.452 0-114.68-17.219-161.137-47.106 8.447.974 16.568 1.299 25.34 1.299 49.055 0 94.213-16.568 130.274-44.832-46.132-.975-84.792-31.188-98.112-72.772 6.498.974 12.995 1.624 19.818 1.624 9.421 0 18.843-1.3 27.614-3.573-48.081-9.747-84.143-51.98-84.143-102.985v-1.299c13.969 7.797 30.214 12.67 47.431 13.319-28.264-18.843-46.781-51.005-46.781-87.391 0-19.492 5.197-37.36 14.294-52.954 51.655 63.675 129.3 105.258 216.365 109.807-1.624-7.797-2.599-15.918-2.599-24.04 0-57.828 46.782-104.934 104.934-104.934 30.213 0 57.502 12.67 76.67 33.137 23.715-4.548 46.456-13.32 66.599-25.34-7.798 24.366-24.366 44.833-46.132 57.827 21.117-2.273 41.584-8.122 60.426-16.243-14.292 20.791-32.161 39.308-52.628 54.253z"/></svg> [@thomas_m_ward](https://twitter.com/thomas_m_ward) <svg xmlns="http://www.w3.org/2000/svg" viewBox="0 0 512 512" class="rfa" style="height:0.75em;fill:currentColor;position:relative;"><path d="M326.612 185.391c59.747 59.809 58.927 155.698.36 214.59-.11.12-.24.25-.36.37l-67.2 67.2c-59.27 59.27-155.699 59.262-214.96 0-59.27-59.26-59.27-155.7 0-214.96l37.106-37.106c9.84-9.84 26.786-3.3 27.294 10.606.648 17.722 3.826 35.527 9.69 52.721 1.986 5.822.567 12.262-3.783 16.612l-13.087 13.087c-28.026 28.026-28.905 73.66-1.155 101.96 28.024 28.579 74.086 28.749 102.325.51l67.2-67.19c28.191-28.191 28.073-73.757 0-101.83-3.701-3.694-7.429-6.564-10.341-8.569a16.037 16.037 0 0 1-6.947-12.606c-.396-10.567 3.348-21.456 11.698-29.806l21.054-21.055c5.521-5.521 14.182-6.199 20.584-1.731a152.482 152.482 0 0 1 20.522 17.197zM467.547 44.449c-59.261-59.262-155.69-59.27-214.96 0l-67.2 67.2c-.12.12-.25.25-.36.37-58.566 58.892-59.387 154.781.36 214.59a152.454 152.454 0 0 0 20.521 17.196c6.402 4.468 15.064 3.789 20.584-1.731l21.054-21.055c8.35-8.35 12.094-19.239 11.698-29.806a16.037 16.037 0 0 0-6.947-12.606c-2.912-2.005-6.64-4.875-10.341-8.569-28.073-28.073-28.191-73.639 0-101.83l67.2-67.19c28.239-28.239 74.3-28.069 102.325.51 27.75 28.3 26.872 73.934-1.155 101.96l-13.087 13.087c-4.35 4.35-5.769 10.79-3.783 16.612 5.864 17.194 9.042 34.999 9.69 52.721.509 13.906 17.454 20.446 27.294 10.606l37.106-37.106c59.271-59.259 59.271-155.699.001-214.959z"/></svg> [thomasward.com](https://thomasward.com) <svg xmlns="http://www.w3.org/2000/svg" viewBox="0 0 384 512" class="rfa" style="height:0.75em;fill:currentColor;position:relative;"><path d="M172.268 501.67C26.97 291.031 0 269.413 0 192 0 85.961 85.961 0 192 0s192 85.961 192 192c0 77.413-26.97 99.031-172.268 309.67-9.535 13.774-29.93 13.773-39.464 0z"/></svg> [MGH Department of Surgery](https://www.massgeneral.org/surgery/) <svg xmlns="http://www.w3.org/2000/svg" viewBox="0 0 384 512" class="rfa" style="height:0.75em;fill:currentColor;position:relative;"><path d="M172.268 501.67C26.97 291.031 0 269.413 0 192 0 85.961 85.961 0 192 0s192 85.961 192 192c0 77.413-26.97 99.031-172.268 309.67-9.535 13.774-29.93 13.773-39.464 0z"/></svg> [MGH SAIIL](https://www.massgeneral.org/surgery/research/surgical-artificial-intelligence-and-innovation-laboratory)